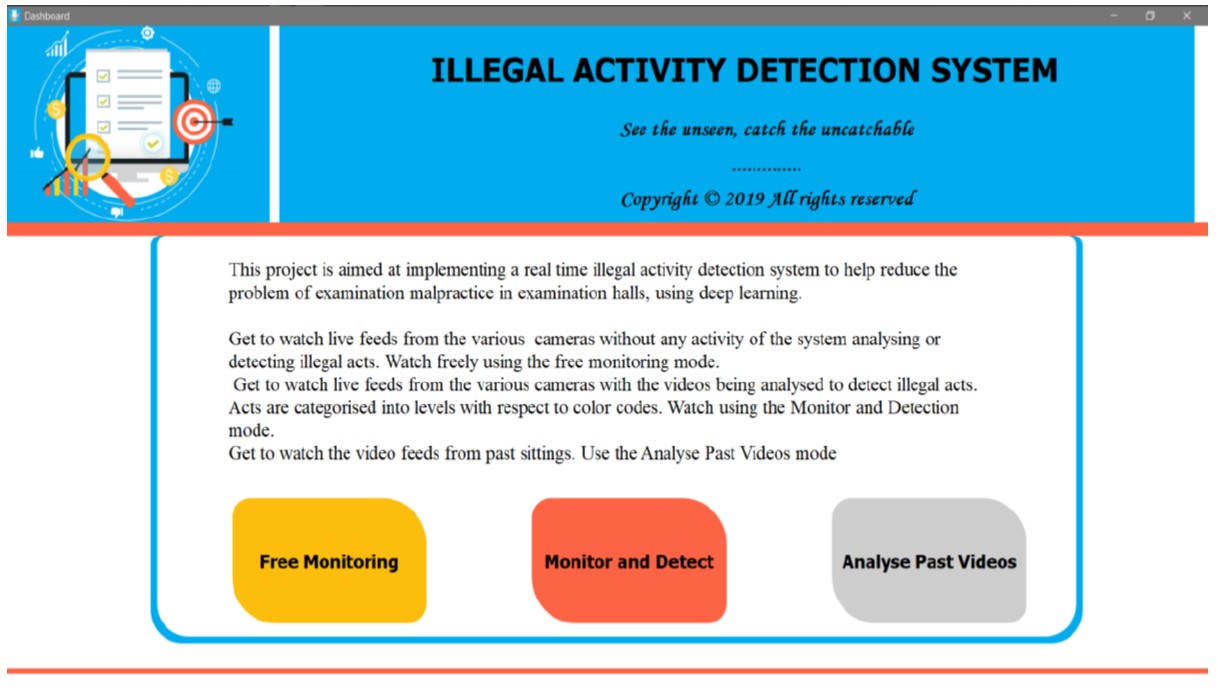
AN ILLEGAL ACTIVITY DETECTION SYSTEM USING DEEP LEARNING
This project is aimed at implementing a real-time illegal activity detection system to help reduce the problem of examination malpractice in examination halls. It seeks to make it effective and efficient for a minimum of one person to monitor feeds from surveillance cameras during examinations with the aid of computer vision. We stacked to only 3 activities that may occur during an exam. A dataset of 456 videos each between 3 to 6 seconds, divided into four classes including normal actions, exchanging of papers, use of foreign material (Mobile phone), looking on another’s work.
Convolutional Neural networks (CNN) which are best at learning spatial and temporal features of an image was used. CNN provided us with two different hybrid architectures; Multi-Layer Perceptron (MLP) and Long Short-Term Memory (LTSM) which are great at classifying global temporal clues. Two models were trained under each network to provide variations. Results obtained from the training showed that CNN + MLP models performed better in detecting illegal activities from our data with accuracies of 79% and 90% whiles CNN + LSTM models network had accuracies of 79% and 89%.
Accuracies recorded from the two-hybrid networks were encouraging, hence these models were used to build desktop application for real-time detection of illegal activities in the examination halls.